In today’s competitive business environment, operational efficiency has emerged as a critical differentiator between thriving organizations and those struggling to maintain market position. For startups and small-to-medium businesses (SMBs), operational challenges can be particularly daunting given their resource constraints and the pressure to establish market presence quickly. Artificial intelligence (AI) technologies present unprecedented opportunities to address these challenges, offering solutions that were previously accessible only to enterprises with substantial technology budgets.
The integration of AI into operational workflows represents more than merely adopting new technology—it constitutes a fundamental reimagining of how work gets done. From automating routine processes to providing predictive insights that inform strategic decisions, AI is transforming operations across industries. This transformation is especially valuable for growing businesses seeking to maximize output while maintaining lean organizational structures.
Identifying High-Impact Operational Applications for AI
The most successful AI implementations begin with clear identification of operational pain points where technology can deliver measurable improvement. Rather than applying AI broadly across the organization, focused deployment targeting specific challenges typically yields superior returns on investment.
Several operational areas consistently demonstrate high potential for AI-driven transformation:
Process Automation and Workflow Optimization
Administrative processes consume substantial resources in most organizations, particularly those in growth phases where systems and procedures are still evolving. AI-powered automation tools can streamline these workflows, reducing manual intervention while improving consistency and reducing error rates.
Consider a professional services firm that implemented intelligent document processing to manage client onboarding. By applying machine learning to automatically extract, categorize, and validate information from client documentation, they reduced processing time by 72% while improving accuracy by 38%. This not only accelerated revenue recognition but enhanced the client experience during a critical relationship-forming period.
Supply Chain Intelligence
For businesses managing inventory or complex supplier networks, AI offers remarkable capabilities for optimization. Predictive analytics can forecast demand fluctuations with increasing accuracy, while machine learning algorithms can identify patterns indicating potential disruptions before they impact operations.
A specialty retailer with 22 locations implemented an AI-driven inventory management system that reduced stockouts by 32% while simultaneously decreasing excess inventory by 27%. The system continuously analyzed sales patterns, seasonal trends, and external factors such as local events and weather forecasts, adjusting ordering recommendations accordingly. This delivered both working capital improvements and revenue gains from increased product availability.
Quality Assurance and Error Reduction
Quality issues create significant operational drag through rework, warranty claims, and customer dissatisfaction. AI-powered visual inspection systems and anomaly detection algorithms can identify potential quality problems with precision that exceeds human capability, particularly for repetitive assessment tasks.
An electronics manufacturer deployed computer vision technology to augment their quality control process, detecting subtle component placement issues that frequently evaded human inspectors. This reduced defect rates by 41% while accelerating inspection time, allowing the company to increase production volume without proportional growth in quality assurance staffing.
Implementation Strategies That Maximize Success
While AI technologies offer substantial operational benefits, successful implementation requires thoughtful planning and execution. Organizations that approach AI deployment strategically consistently achieve better outcomes than those pursuing technology adoption without clear frameworks.
Start with Defined Success Metrics
Effective AI implementations begin with explicit definition of the outcomes they’re designed to achieve. These metrics should directly connect to business objectives such as cost reduction, cycle time improvement, or quality enhancement. Without clear success criteria, organizations struggle to evaluate results and justify continued investment.
The metrics should include both implementation milestones (e.g., system deployment completion, user adoption rates) and business impact measures (e.g., processing time reduction, error rate decreases, cost savings). Establishing baseline measurements before implementation provides essential context for assessing improvement.
Address Data Quality Early
AI systems depend fundamentally on data to develop insights and guide decisions. Poor data quality inevitably leads to poor results, regardless of how sophisticated the underlying algorithms may be. Successful implementations typically include data assessment and remediation phases before full AI deployment.
This preparation involves evaluating completeness, accuracy, consistency and accessibility of relevant data sources. Organizations often discover that improving data infrastructure delivers operational benefits even before AI capabilities are fully implemented, creating early wins that build implementation momentum.
Develop Human-AI Integration Models
The most effective operational AI applications complement human capabilities rather than attempting to replace them entirely. Well-designed systems automate routine elements while escalating exceptions requiring judgment to appropriate personnel, creating more engaging roles focused on higher-value activities.
A financial services organization implemented an AI-powered claims processing system that automatically handled straightforward cases while routing complex scenarios to specialists. This not only accelerated processing times but improved employee satisfaction by allowing staff to concentrate on challenging work requiring their expertise rather than routine paperwork.
Overcoming Implementation Challenges
Despite their potential benefits, AI implementations frequently encounter obstacles that can undermine their effectiveness. Addressing these challenges proactively significantly improves success rates.
Building Internal Capability
While vendor partners often provide implementation support, organizations need internal expertise to guide these initiatives effectively. This doesn’t necessarily require hiring data scientists or AI specialists—particularly for smaller organizations—but does demand developing sufficient understanding to make informed decisions about technology selection and deployment.
Many successful organizations designate “AI champions” who receive additional training and serve as bridges between technical implementation teams and operational users. These individuals typically combine domain expertise with interest in technology, allowing them to identify high-value applications and support effective change management.
Managing Change and Adoption
Technology implementation represents only part of the AI journey. Achieving full benefits requires changes in how people work—often significant ones that may encounter resistance. Effective change management strategies acknowledge concerns while clearly communicating benefits, both organizational and individual.
Involving end users early in the implementation process improves both system design and adoption rates. Users who contribute to defining requirements and testing prototypes develop stronger ownership of the solution, becoming advocates rather than resisters during broader deployment.
Ensuring Responsible AI Use
As AI capabilities become increasingly integrated into operations, questions of bias, transparency, and accountability grow correspondingly important. Organizations implementing these technologies must establish governance frameworks that ensure responsible use while maintaining operational efficiency.
This governance should address data privacy, algorithmic transparency, and monitoring for unintended consequences or biases. Rather than viewing these considerations as constraints, forward-thinking organizations recognize that responsible implementation builds trust with both customers and employees, creating sustainable competitive advantage.
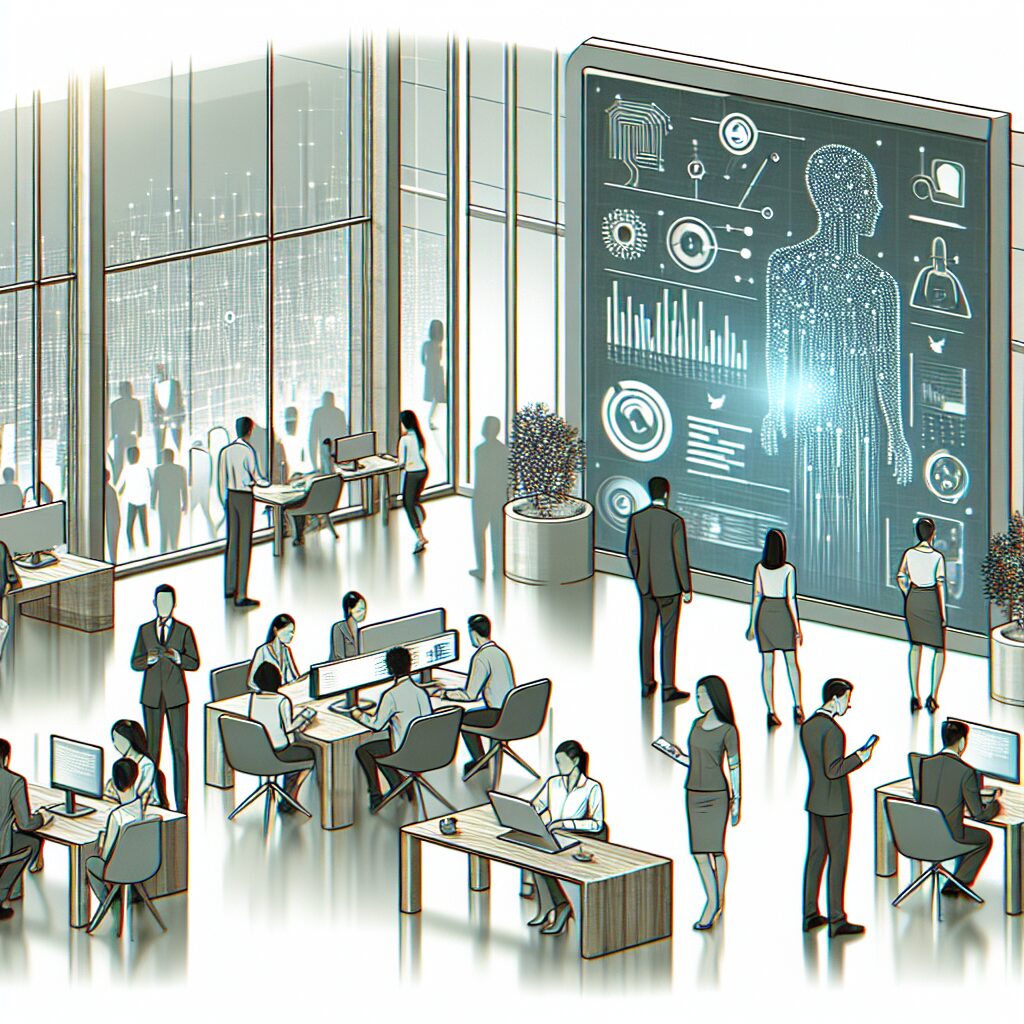
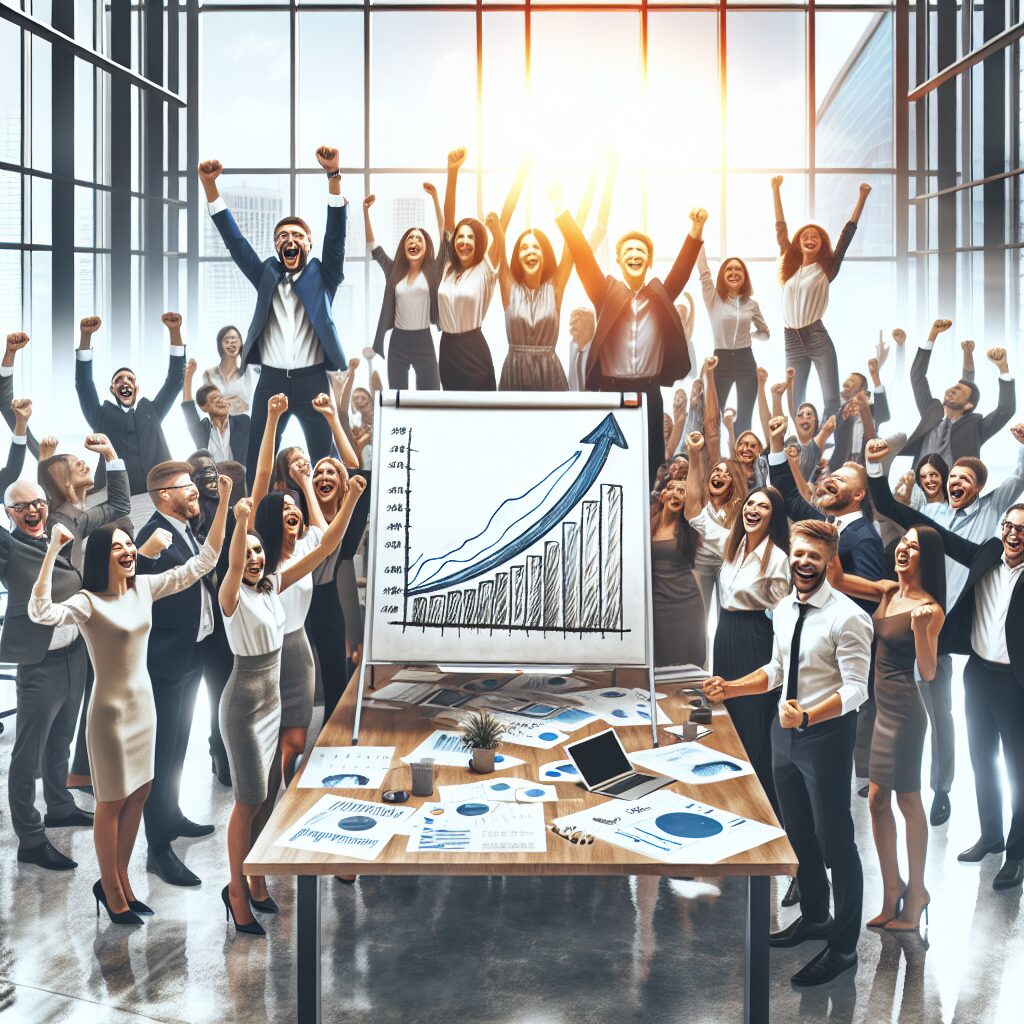
Scaling Success: From Pilot to Enterprise Integration
Organizations that achieve success with initial AI implementations often struggle to scale these successes across operations. Moving from isolated applications to integrated capabilities requires additional considerations beyond those relevant to pilot programs.
Creating Platform Approaches
Rather than implementing disparate AI solutions for individual challenges, mature organizations develop platform approaches that provide consistent capabilities across functions. These platforms typically include shared data repositories, reusable models, and consistent governance frameworks.
This approach not only reduces technical overhead but accelerates implementation of new applications by leveraging existing components. A healthcare provider that initially implemented AI for appointment scheduling subsequently expanded to referral management and preventive care outreach, achieving the latter implementations in one-third the time required for the initial application through platform reuse.
Developing Centers of Excellence
As AI capabilities mature within organizations, centralizing expertise in centers of excellence can accelerate adoption while ensuring consistent quality and governance. These centers typically combine technical specialists with business process experts, creating cross-functional teams that can effectively bridge technology and operations.
The centers serve as internal consultancies, helping functional areas identify opportunities, implement solutions, and measure outcomes. This model preserves domain knowledge within business units while providing specialized expertise that would be inefficient to replicate across the organization.
Looking Forward: Emerging Opportunities
As AI technologies continue to evolve, new operational applications emerge regularly. Several developing capabilities show particular promise for addressing persistent operational challenges:
Conversational AI for Internal Service Delivery
Advanced natural language processing is enabling increasingly sophisticated conversational interfaces for internal service functions like IT support, HR assistance, and administrative help. These systems can handle a growing proportion of routine inquiries while continuously learning from interactions, improving their capabilities over time.
Operational Digital Twins
Digital twin technology—creating virtual replicas of physical operations—enables sophisticated simulation and scenario planning. Organizations can test process changes virtually before implementing them physically, reducing disruption while improving outcomes. This capability is expanding from manufacturing environments to service operations, offering new opportunities for operational optimization.
Autonomous Decision Systems
While current AI implementations typically augment human decision-making, autonomous systems capable of making and executing routine operational decisions within defined parameters are emerging in areas like resource allocation, scheduling, and basic customer service. These systems promise to further accelerate operations while maintaining consistent quality.
Key Takeaways
For startups and SMBs navigating competitive markets with constrained resources, AI technologies offer unprecedented opportunities to enhance operational efficiency and effectiveness. By identifying high-impact applications, implementing strategically, addressing challenges proactively, and establishing frameworks for scaling success, these organizations can achieve operational capabilities that were previously available only to much larger enterprises.
The most successful organizations approach AI not as a technological initiative but as a business transformation enabled by technology. They focus relentlessly on measurable outcomes, involve stakeholders throughout the implementation process, and establish governance frameworks that ensure responsible use. This balanced approach delivers sustainable operational improvements that create lasting competitive advantage.
As AI capabilities continue to evolve, the operational possibilities will expand accordingly. Organizations that establish strong foundations now—building internal expertise, developing platform approaches, and creating effective human-AI integration models—will be best positioned to capitalize on these emerging opportunities, maintaining operational excellence in increasingly dynamic markets.
If you would like to learn more about how your company can take advantage of the improvements in AI, then reach out the form below:
Leave a Reply